The neuro-expert system "Kardionet"
allows to diagnose cardiovascular diseases, predicts their progression over 5, 10, 15 years, or more, and tailors optimal treatment and prevention courses considering patients’ individual characteristics.
Start the examinationThe creation history of the intelligent diagnostic and prognostic system KARDIONET
This story began in 1998, i.e. more than 20 years ago.
Professor Leonid Yasnitsky was engaged in the development of a new training course "Artificial Intelligence", which he had to teach students instead of the deceased Yuri Devingtal. Together with the workload hi inherited an extensive library of books published before 1980, i.e. before the beginning of perestroika, the collapse of the USSR and the degradation of Soviet science. These were books on the mathematical and philosophical foundations of Artificial Intelligence.
It should be noted that at that time the stigma of bourgeois pseudo-science from Cybernetics was already removed, but the attitude to Artificial Intelligence was as to some useless philosophical science. There were endless debates and discussions on the topic "Can a machine think?". And in some books it was very categorically stated that neural networks are a dead-end scientific direction.
It all started with the fact that L.Yasnitsky did not believe in this statement. He was delighted with the experiments of Frederick Rosenblatt on the recognition of letters of the Latin alphabet. It was surprising that the neural network, invented by F. Rosenblatt, imitated the processes taking place in the brain. In addition, the knowledge in it is not laid in advance, as in a conventional computer program, and acquired during the training method of "reward – punishment", ie, as it is practiced in the training of children.
It was a brilliant attempt to create an artificial brain. It was clear that the idea of "machine learning" opens up tremendous opportunities for solving a wide range of practical problems!
Using F.Rosenblatt's method, L.Yasnitsky compiled a computer program and tried to teach it the multiplication table.
It worked!
Moreover, the neural network, having learned from some examples, successfully coped with such examples, which it was not taught. This property – to give correct answers to unfamiliar questions, in the theory of artificial intelligence is called the property of generalization. It is thanks to the property of generalization (by the way, it is inherited from the brain) it became clear that neural networks are not a dead end, and not a toy for philosophers, and technology, for which it was possible to predict the great prospects of practical application.
The first large order for the use of the new method agreed to give the Perm aviation plant. It was necessary to solve an important practical problem – to create an intelligent system designed for early diagnosis of aircraft engines. From the plant to the University transmitted information about the defects and malfunctions found in the disassembly of aircraft engines removed from the aircraft. At the same time, we were given the values of the operating parameters of aircraft engines, which were measured during the flights. Among these parameters were: engine age, temperature at various points, pressure, pulsations, etc. It was like the parameters of a patient being diagnosed by a doctor.
Thus, a training set of examples was formed – records for each engine, including parameters characterizing its operation during flights (input parameters) and information about the detected faults (output parameters). The neural network was successfully trained and during the tests it made the correct diagnoses. It revealed the malfunctions of aircraft engines prior to their disassembly (before opening). Moreover, the neural network revealed even such faults that conventional engineering methods could not be detected.
The next project was the development of neural lie detectorended in its introduction of the practice of activities of OOO "Polyconius" – the world leader in the market of polygraph products and services. The principle of creating a neural network polygraph was similar. Under the leadership of the chief polygraph examiner of the Perm region Colonel Askold Markovich Petrov collected data from his polygraph surveys: parameters characterizing the object of study (human): pulse rate, pressure, respiratory parameters, electrical conductivity of the skin, electrocardiogram, etc., as well as the diagnosis made by the polygraph examiner – the truthful answer was given by the Respondent at the time of removing the parameters, or false.
At the same time, experimental work was carried out to create software tools for the generation and training of neural networks. Started by Leonid Yasnitsky, Alexey Shveyev, Sergey Burdin, these software tools for fifteen years have been constantly improved and supplemented by Fedor Cherepanov new modern (including author) subroutines that allow you to create neural networks that are best suited for each task. Using Application package NSIM-5.0 (it is freely available on the website www.lbai.ru) dozens of intellectual systems have been created in the field of Economics, business, industry, political science, sociology, psychology, criminology, sports, etc., which are devoted to hundreds of our scientific publications. Thanks to this, the Perm branch of the Scientific Council of the Russian Academy of Sciences on the methodology of artificial intelligence now has a serious scientific priority, and the head of the branch L.Yasnitsky since 2017 according to RSCI is in the TOP 100 most cited Russian scientists in the field of Cybernetics and Informatics.
But one day, the project Manager L.Yasnitsky with a heart attack on the ambulance was taken to the Fourth clinical hospital in c.Perm. There he met a wonderful doctor, PhD Andrei Dumler and convinced him to develop a questionnaire to collect statistical information about his patients. The questionnaire contained seven sections:
- Passport part.
- Complaints.
- History of the disease.
- The story of the life of the patient.
- Objective (physical) research.
- Electrocardiography.
- Additional research methods (laboratory, instrumental).
The system was trained to detect the following diagnoses:
- Myocardial Infarction.
- Angina is stable.
- Angina is unstable.
- Hypertensive disease.
- Cardiac arrhythmias and conduction.
- Acute left ventricular failure.
- Congestive Heart Failure.
- Pulmonary embolism.
Statistical data collection and neural network training experiments began in 2005 with the help of a small group of enthusiasts. From 2009 to 2012, these works continued to be carried out with the financial support of a grant From the Government of the Perm region. Employees of JSC "Information and computer systems" (IVS), Perm state medical University named after academician E. A. Wagner, Perm state national research University, Perm state humanitarian and pedagogical University were involved in.
By 2012, neural networks have already been trained on the personal data of more than a thousand cardiac patients. However, although the accuracy of diagnosis when testing the neural network system ranged within acceptable limits, while trying the trial operation of the system has identified cases of incorrect diagnoses.
2013 – 2015 can be called a period of rethinking, detection and correction of errors. As a result of long discussions of modeling methods and test results of the intelligent system, the causes of erroneous diagnosis were established. Between mathematicians and doctors, finally, was found "common language."
During this period of the time, a neural network was invented, specifically designed to search for statistical information emissions – errors that inevitably occur in large amounts of data. The following year was devoted exclusively to the identification and correction of erroneous information in the training sets with the help of a new neural network tool, which was used in close contact with medical experts. From 2016 to 2018, work on the project was carried out with the financial support of the Russian Foundation for basic research.
By 2018 was cleared of all claims of the doctors practical. The intelligent KARDIONET system has been successfully tested in the Fourth clinical hospital in Perm.
In the same year, in addition to the ability to diagnose diseases, the system was taught to predict the appearance and development of diseases for 5, 10, 15 or more years. This, in turn, made it possible to select the optimal lifestyle for each patient, as well as to optimize the courses of prevention and treatment of cardiovascular diseases.
Comparing the results of our project with the world achievements, it should be noted that there are already many neural network medical systems in the world that can diagnose a wide variety of diseases. There are reports that mention the terms "disease prognosis", "disease prediction", "optimization of treatment and prevention". But, as shown by the analysis of world literature, performed in our scientific article, with a closer examination of the texts of publications, it turns out that these terms are used only in the narrow sense of the word – as "the outcome of the disease", "survive – will not survive", "what percentage of patients will survive", "whether the patient will go back to the doctor", or as "diagnosis, predicted in the uncertain future." And by "optimization" sometimes means optimizing the cost of treatment. Information about the real use of the apparatus of neural networks for modeling diseases as processes that develop over time, especially – to control these processes, we could not find anywhere.
The uniqueness of our project is that we have learned to create dynamic computer models of patients using artificial intelligence methods. We learned how to perform virtual computer experiments on computer models – virtually increase the age of the patient and observe what diseases he may develop in the future. We have learned to virtually change the patient's lifestyle and try different courses of prevention and treatment. Watching on the computer screen, what it will lead to in the near and long term, we have learned to choose the best way of life for the patient, optimal drugs, optimal courses of prevention and optimal treatment of diseases.
But, there are natural questions: Why in the world still nobody does it? Why are there no such intelligent systems on the market of medical software?
Answering these questions, it should be noted that we build our scenario forecasts using the method of freezing the input parameters. This means that we virtually change one of the input parameters, leaving the other input parameters unchanged. But, the problem is that human parameters have complex correlations. For example, with increasing age in humans there are changes in the electrocardiogram, changes in the results of echocardiography and biochemical analyzes, etc. However, such dependencies are usually unknown in advance, and therefore it is very difficult to take them into account when building neural network models. This problem was the "stumbling block" for scientists. That is why in the world scientific literature it is impossible to find examples of the use of neural networks for full scenario forecasting of diseases, i.e. – for modeling diseases as processes developing in time.
To overcome this "stumbling block" the authors of this project in scientific articles proposed a way to ideologically combine the capabilities of two technologies of artificial intelligence: neural networks and expert systems. We proposed an original algorithm that allows us to adjust the results of neural network scenario prognosis of disease development with the help of knowledge inherent in the international scale SCORE, and other techniques that are the Know-How of our project.
Now the KARDIONET system, performing long-term forecasts, models our psychological ideas about how a person makes decisions. First, his decisions are formed under the influence of intuition, emotions, experience. According to the basic hypotheses of Neuroinformatics (McCullock, Pitts, Rosenblatt), these preliminary emotional and intuitive solutions are produced as a result of the computational activity of neurons in the human biological neural network. However, these preliminary decisions (including diagnoses and projections) are then adjusted. In the final form, decisions are made by a person after thinking, i.e. with the use of expert knowledge – the rules and laws known to a person about the subject area or the environment in which he lives.
Following this idea, we were able to create an intelligent system KARDIONET, capable not only to put the current diagnosis of diseases, but also to carry out forecasts of their appearance and development for long periods of time.
Since 2017, the authors of the project have begun to search for opportunities for a wider introduction of KARDIONET in medical practice. KARDIONET system was demonstrated at medical scientific conferences and meetings in Perm, Yekaterinburg, Tyumen, Moscow, Budva (Montenegro), etc.
The report made in Moscow in 2017 at the XII National Congress of therapists, was highly appreciated by the Chairman of the Congress, the President of the Russian scientific medical society of therapists, academician of RAS A.I.Martynov, who agreed to become a mentor of the project and gave a letter of recommendation.
With similar success, the presentation and demonstration of KARDIONET at the II International medical investment forum MMIF-2018 were held, where the relevance of the project was confirmed by business representatives, including the moderator of the session, Vice-President of the SKOLKOVO Foundation Mr. K.V.Kaem.
In 2019, KARDIONET was demonstrated and discussed at the «Round table»within the framework of the Fourth all-Russian scientific and practical conference "Artificial intelligence in solving urgent social and economic problems of the XXI century". Participants of the Round table – authoritative scientists in the field of medicine, philosophy, psychology, mathematical modeling, came to the conclusion that with the advent of dynamic intelligent systems like KARDIONET, capable not only to diagnose medical diseases, but also to predict their occurrence and development, opens the possibility of radical solution to centuries-old ethical problem "doctor-patient". Now doctors, before prescribing medicines, courses of prevention and treatment of patients, will observe, check and optimize their action not on the patients themselves, but on mathematical models of patients. Thus, "conditions are created for the transition of medical science and practice to a qualitatively new level corresponding to the time».
The results of the demonstration of the KARDIONET system, as well as its discussions and discussions at the Round table were published in the Conclusions of the conference.
Why trust us
- The diagnosis and prognosis of the appearance and development of diseases is performed by artificial intelligence trained on the histories of more than 3,000 cardiac patients.
- The KARDIONET system takes into account a wide range of individual parameters of the human body, including genetic and hereditary factors, conditions and lifestyle, and more.
- KARDIONET has successfully passed comprehensive testing and trial operation in a number of medical centers.
- KARDIONET was created by a team of experienced specialists in the field of medicine and artificial intelligence.
- The method of creating KARDIONET published by the authors in a number of reputable peer-reviewed journals from the database RSCI, VAK, SCOPUS, etc.
- We were recognized by the medical community headed by the President of the Russian scientific medical society of therapists, academician of RAS A.I.Martynov:
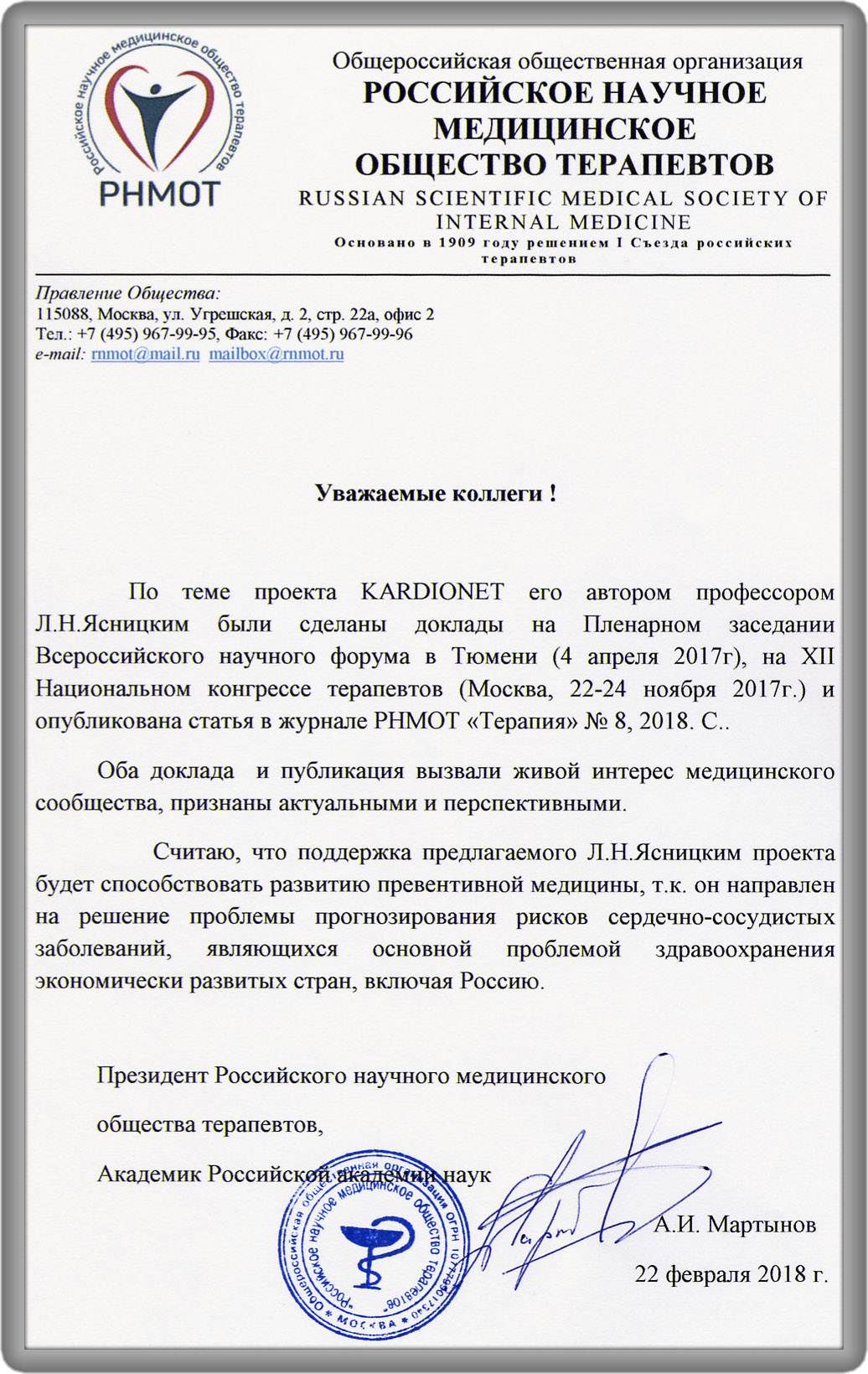
Scientific publication
- Ясницкий Л.Н. Введение в искусственный интеллект. М.: Издательский центр «Академия», 2005. – 176с.
- Ясницкий Л.Н. Интеллектуальные информационные технологии и системы / Пермский ун-т. Пермь, 2007. 271с.
- Ясницкий Л. Н., Черепанов Ф.М., и др. Пермская научная школа искусственного интеллекта и ее инновационные проекты. 2-е изд. Москва-Ижевск: НИЦ «Регулярная и хаотическая динамика», 2008. 75с. (Скачать)
- Ясницкий Л.Н., Думлер А.А., Полещук А.Н., Богданов К.В., Черепанов Ф.М. Нейросетевая система экспресс-диагностики сердечно-сосудистых заболеваний // Пермский медицинский журнал. – 2011. – Т.28. – №4. – С. 77-86.
- Ясницкий Л.Н., Думлер А.А., Богданов К.В., Полещук А.Н., Черепанов Ф.М., Макурина Т.В., Чугайнов С.В. Диагностика и прогнозирование течения заболеваний сердечно-сосудистой системы на основе нейронных сетей // Медицинская техника. 2013. № 3. С. 42-44. DOI: 10.1007/s10527-013-9359-0. (Скачать)
- Yasnitsky L.N., Dumler A.A., Bogdanov K.V., Poleschuk A.N., Cherepanov F.M., Makurina T.V., Chugaynov S.V. Diagnosis and Prognosis of Cardiovascular Diseases on the Basis of Neural Networks // Biomedical Engineering. 2013. Vol. 47. No 3. Pp. 160-163. DOI: 10.1007/s10527-013-9359-0. (Скачать)
- Yasnitsky L.N., Dumler A.A., Poleshchuk A.N., Bogdanov C.V., Cherepanov F.M. Artificial Neural Networks for Obtaining New Medical Knowledge: Diagnostics and Prediction of Cardiovascular Disease Progression // Biology and Medicine. 2015. 7(2), BM-095-15, 8 pages. (Скачать)
- Ясницкий Л.Н. Интеллектуальные системы : учебник. М.: Лаборатория знаний, 2016. 221 с. (Скачать)
- Ясницкий Л.Н. Новые возможности применения методов искусственного интеллекта в медицине: диагностика, прогнозирование и моделирование развития заболеваний сердечно-сосудистой системы // XII Национальный конгресс терапевтов (сборник тезисов) (г. Москва, 22–24 ноября 2017 г.). – Москва, 2017. С. 153.
- Черепанов Ф.М., Ясницкий Л.Н. Нейро-экспертная система диагностики и прогнозирования рисков сердечно-сосудистых заболеваний. Свидетельство о государственной регистрации программы для ЭВМ № 2017662410. Заявка Роспатент № 2017619552. Зарегистрировано в Реестре программ для ЭВМ 07 ноября 2017г. (Скачать)
- Yasnitsky L.N., Dumler A.A, Cherepanov F.M. The Capabilities of Artificial Intelligence to Simulate the Emergence and Development of Diseases, Optimize Prevention and Treatment Thereof, and Identify New Medical Knowledge // Journal of Pharmaceutical Science and Research. 2018. Vol. 10(9). Pp. 2192-2200. (Скачать)
- Ясницкий Л.Н., Черепанов Ф.М. Нейроэкспертная система диагностики, прогнозирования и управления рисками сердечно-сосудистых заболеваний // Прикладная математика и вопросы управления. 2018. № 3. С. 107-126. DOI: 10.15593/2499-9873/2018.3.08. (Скачать)
- Yasnitsky L.N., Dumler A.A., Cherepanov F.M. (2019) Dynamic Artificial Neural Networks as Basis for Medicine Revolution. In: Antipova T., Rocha A. (eds) Digital Science. DSIC18 2018. Advances in Intelligent Systems and Computing, vol 850, pp. 351-358. Springer, Cham. (Proceedings of the 2018 International Conference on Digital Science (DSIC’18). Budva, Montenegro, October 19 – 21, 2018). DOI: (Скачать)
- Черепанов Ф.М. Методы повышения эффективности нейросетевых рекомендательных систем в условиях ограниченных объемов выборок со сложными корреляционными связями (на примере диагностики и прогнозирования сердечно-сосудистых заболеваний человека). 2019. Диссертация на соискание ученой степени кандидата технических наук. (Скачать)
Media about us
TV Program "Vesti Perm"
Video about the Congress of therapists
Certificates
Rospatent certificates for neuropackages intended for generation and training of neural networks
Rospatent certificate on a demonstration prototype KARDIONET
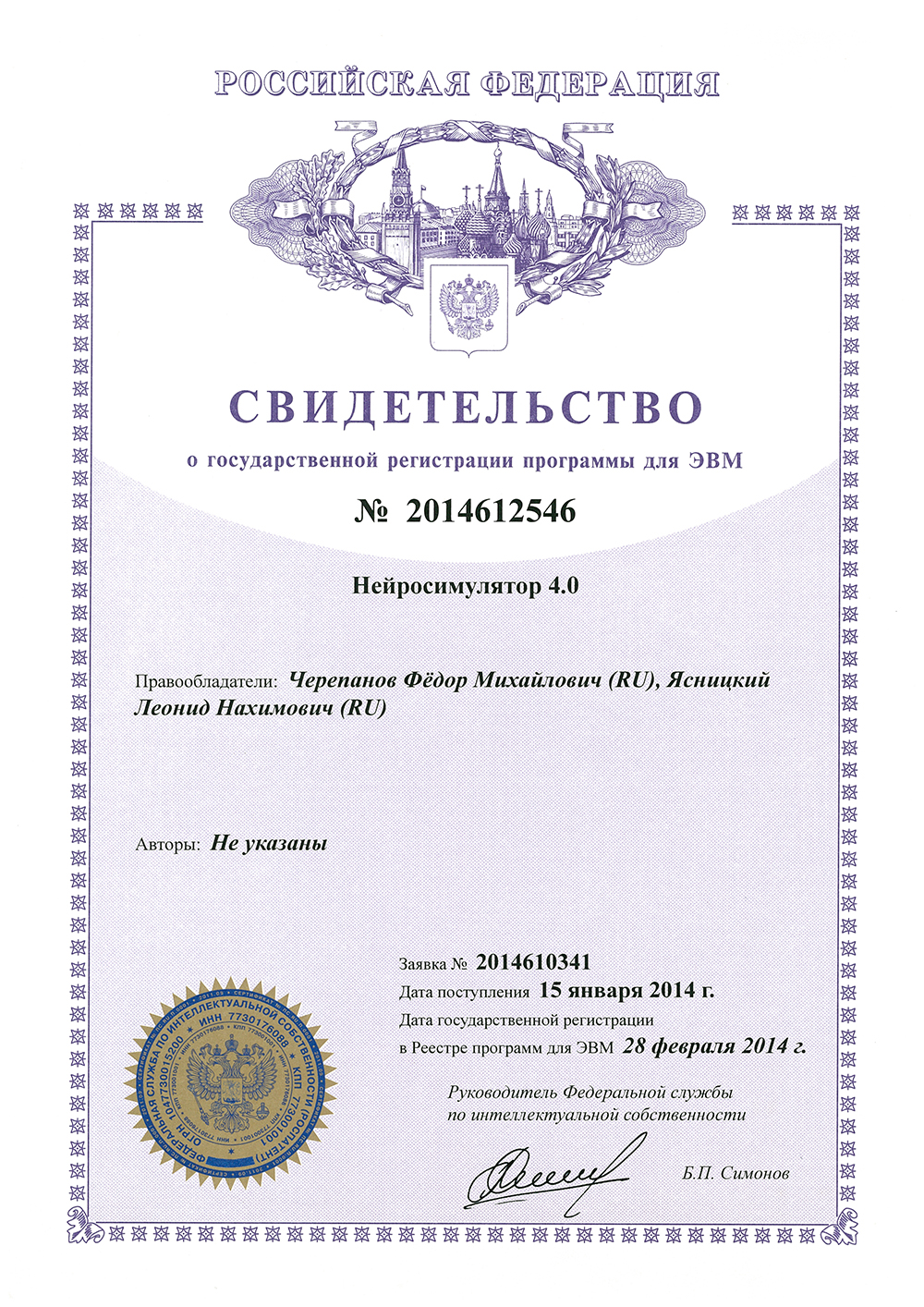
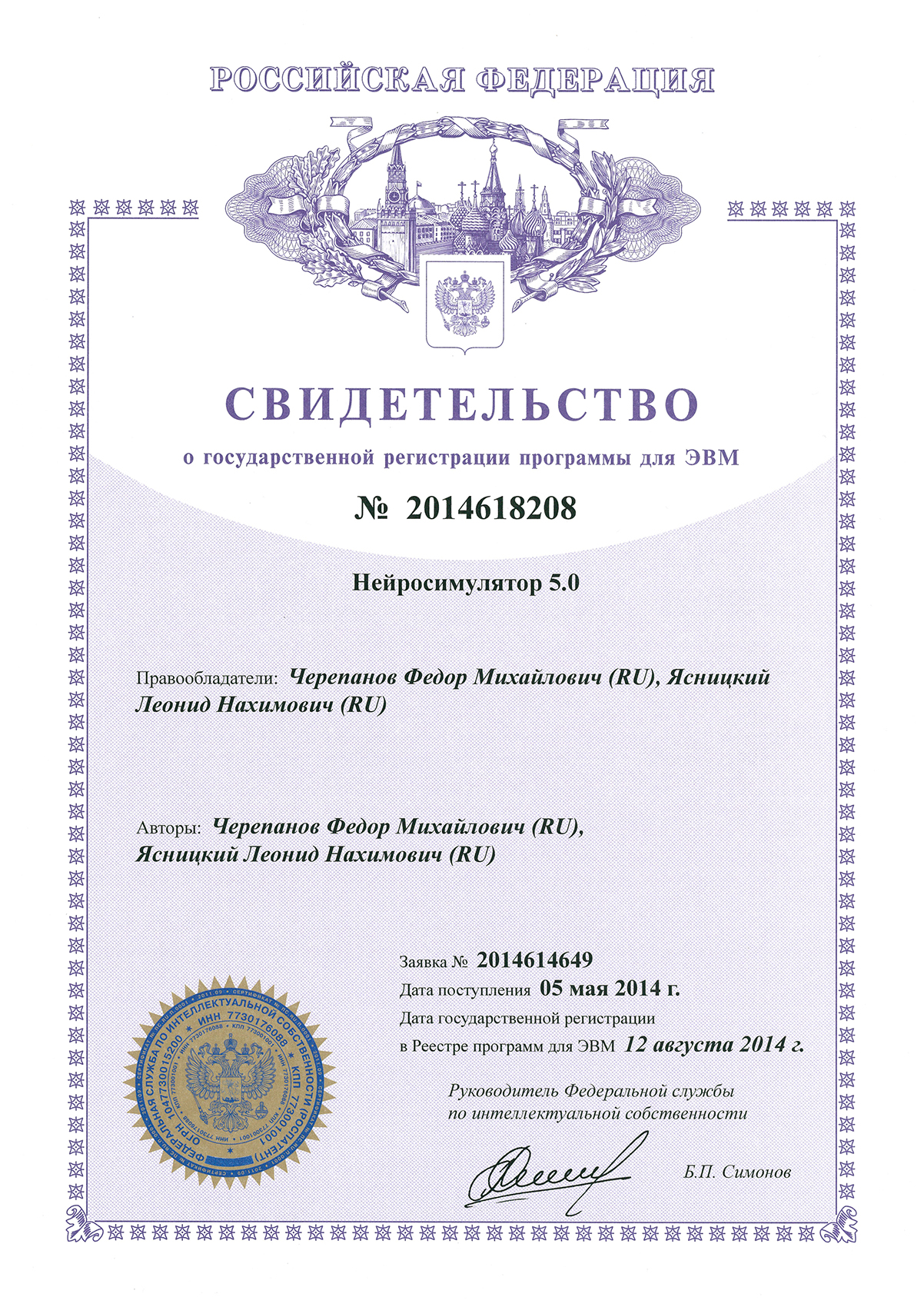
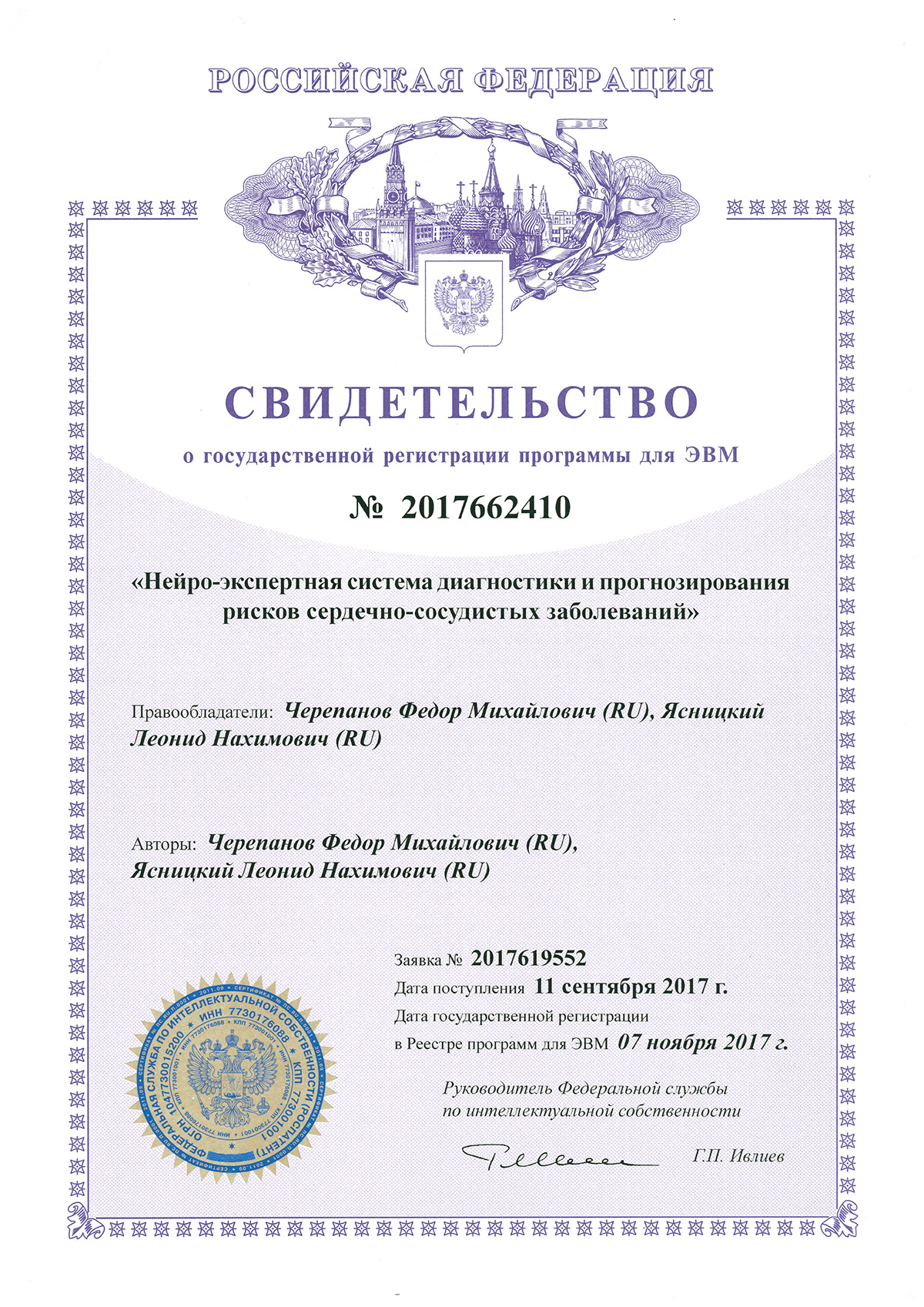
Team
Supervisor: Leonid Yasnitsky, Doctor of technical Sciences, Professor, Chairman of the Perm branch of the Russian Academy Scientific Council on artificial intelligence
Head of doctors Department: Andrey Dumler, Candidate of medical Sciences, associate Professor.
Head of programmers Department: Fedor Cherepanov, PhD of technical Sciences, senior lecturer.
Operational management: Vitaly Yasnitsky, MBA (GSOM "MIRBIS"), candidate of economic Sciences, associate Professor.
Mentor: Anatoly Martynov, President of the Russian scientific medical society of therapists, Academician of RAS, MD, Professor.
Contacts
Тел.: +7 (342) 27-16-168, +7 (912) 07-04-387
email: yasn@psu.ru